Best Tech Stocks Performance Report By I Know First Evaluation
Executive Summary
The purpose of this report is to present the results of live forecast performance evaluation for I Know First AI Algorithm, specifically for Big Tech Stocks. The following results were observed when signal and predictability filters were applied to pick the best-performing stocks out of the most predictable ones. The period under evaluation is from 21st November 2018 to 26th January 2020. The corresponding returns distribution of stock filters for Big Tech stock universe are shown below:
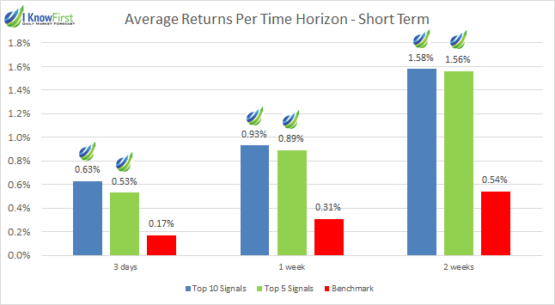

Big Tech Stocks Package Highlights:
- Both Top 10 and Top 5 signals surpass the 24% mark in the one-year time horizon
- There is a clear increasing trend for returns improvement with the time horizon increase for both Top 10 and Top 5 stocks
- Both Top 10 and Top 5 stocks consistently out-perform the benchmark
The above results were obtained based on forecasts’ evaluation over the specific time period using a consecutive filtering approach – by predictability, then by signal, to give an overview of the forecasting capabilities of the algorithm for the specific stock universe.
About the I Know First Algorithm

The I Know First self-learning algorithm analyzes, models, and predicts the stock market. The algorithm is based on Artificial Intelligence (AI) and Machine Learning (ML) and incorporates elements of Artificial Neural Networks and Genetic Algorithms.
The system outputs the predicted trend as a number, positive or negative, along with a wave chart that predicts how the waves will overlap the trend. This helps the trader to decide which direction to trade, at what point to enter the trade, and when to exit. Since the model is 100% empirical, the results are based only on factual data, thereby avoiding any biases or emotions that may accompany human derived assumptions.
The human factor is only involved in building the mathematical framework and providing the initial set of inputs and outputs to the system. The algorithm produces a forecast with a signal and a predictability indicator. The signal is the number in the middle of the box. The predictability is the number at the bottom of the box. At the top, a specific asset is identified. This format is consistent across all predictions.
Our algorithm provides two independent indicators for each asset – Signal and Predictability.
The Signal is the predicted strength and direction of the movement of the asset. Measured from -inf to +inf.
The predictability indicates our confidence in that result. It is a Pearson correlation coefficient between past algorithmic performance and actual market movement. Measured from -1 to 1.
You can find a detailed description of our heatmap here.
The Stock Picking Method
The method in this evaluation is as follows:
We take the top X most predictable assets and, from them, we pick the top Y highest signals.
By doing so we focus on the most predictable assets on the one hand, while capturing the ones with the highest signal on the other.
For example, a top 30 predictability filter with a top 10 signal filter means that on each day we take only the 30 most predictable assets, and then we pick from them the top 10 assets with the highest absolute signals.
We use absolute signals since these strategies are long and short ones. If the signal is positive, then we buy and, if negative, we short.
The Stock Market Forecast Performance Evaluation Method
We perform evaluations on the individual forecast level. It means that we calculate what would be the return of each forecast we have issued for each horizon in the testing period. Then, we take the average of those results by strategy and forecast horizon.
For example, to evaluate the performance of our 1-month forecasts, we calculate the return of each trade by using this formula:

This simulates a client purchasing the asset based on our prediction and selling it exactly 1 month in the future.
We iterate this calculation for all trading days in the analyzed period and average the results.
Note that this evaluation does not take a set portfolio and follow it. This is a different evaluation method at the individual forecast level.
The Hit Ratio Method
The hit ratio helps us to identify the accuracy of our algorithm’s predictions.
Using our Daily Forecast and Global Model asset filtering, we predict the direction of the movement of different assets. Our predictions are then compared against actual movements of these assets within the same time horizon.
The hit ratio is then calculated as follows:
For instance, a 90% hit ratio for a top 30 predictability filter with a top 10 signal filter would imply that the algorithm correctly predicted the price movements of 9 out of 10 assets within this particular set of assets.
The Benchmarking Method – S&P 500 Index
In order to evaluate our algorithm’s performance in comparison to the US market, we used the S&P 500 as a benchmark.
The S&P 500 measures the stock performance of the largest 500 companies by market cap listed on different stock exchanges in the United States. It is one of the most followed equity indices and is frequently used as the best indicator for the overall performance of US public companies, and the US market as a whole. S&P 500 is a capitalization-weighted index, the weight of each company in the index is determined based on its market cap divided by the aggregate market cap of all the S&P 500 companies.
For each time horizon, we compare the S&P 500 performance with the performance of our forecasts after the filtering processes described above. For evaluating the performance of the forecasts that are filtered based on the Global Model method, we compare those with the average S&P 500 performance only when the Global Model filtering resulted in forecasts for S&P 500 companies. For example, if after our Global filtering the model recommended buying a given asset and hold it three days on five different occasions, the S&P 500 performance we will use as a benchmark will be the average of returns only in these five intervals of three days.
Stock Universe Under Consideration – Big Tech Stock Market
In this report, we conduct testing for Big Tech stocks that I Know First cover by its algorithmic forecast in the “Big Tech stocks” package. Some Big Tech Stocks covered are Apple Inc., NVIDIA Corporation, Micron Technology, Inc. and Applied Materials, Inc. The period for evaluation and testing is from 21st November 2018 to 26th January 2020. During this period, we were providing our clients with daily forecasts for Big Tech stocks and the time horizons which we evaluate in this report are 4 periods spanning from 3 days to 1 month.
Evaluating the Signal Indicator for Big Tech
In this section, we will demonstrate how our stock-picking method can improve if we add the signal indicators.

Considering Table 1, we can see that by considering the Big Tech stocks universe, the percentage increases when signal strength is applied. This occurs in all time horizons: from 3 days to 1 year. Also, we can see a significant jump from the returns when the time horizon hits two weeks when comparing to returns of 3 days and 1 week. Also, when applying the signal strength, the return percentage is higher than the benchmark in every time horizon.
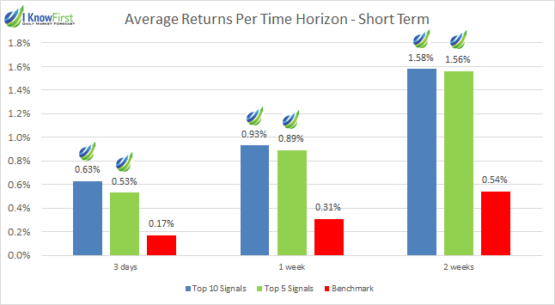

When we compare Figure 1 with Figure 2, it is possible to observe the same situation, when the signal strength applied make the returns consistently higher than the benchmark, with the 3 months horizon being the exception. Also, both Top 10 and Top 5 Signals got the same return by our longest time horizon, with a 24.22 percentage.

From Figure 3, we can observe the same pattern from both Top 10 and Top 5 Signals. Even though the similarities, the Top 10 Signals had a bigger percentage in some time horizons, especially in the longer ones. Besides, both top signals give more than 58% on any time horizon observed, with their peak coming from 1 year by an 84% and 83% hit ratio for the top 10 and top 5 signals, respectively.
Conclusion
This analysis represents the outperformance of the forecasts predicted by the algorithm of I Know First for Big Tech Stocks between 21st November 2018 and 26th January 2020. The evaluation was made comparing the average returns per time horizon and the hit ratio per time between the Top 10 Signals, the Top 5 Signals and S&P 500, the benchmark.
When we filter by signal strength and apply the predictability indicator, we can observe results with better performance than the benchmark of the Big Tech stocks. Except for the 3 months horizon, all the other returns are higher than the benchmark. That performance comes from both Top 10 and Top 5 Signals when we look to the short and long-term numbers. When considering the hit ratio perspective, we saw that the Top 10 Signals got a higher return in each of the time horizons compared to the Top 5 Signals, even though both of them follow the same trend.
All in all, an investor can improve his investments by adding Big Tech stocks to his portfolio when considering the I Know First predictability indicator.